Understanding Research and Analysis: Key Insights for Effective Analysis
Research and analysis are fundamental components of acquiring knowledge in various fields, including public health, social sciences, and market research. This article delves into the definitions, importance, and roles of analysts in the research process, highlighting how effective analysis can lead to informed decision-making and improved outcomes. By understanding these concepts, readers can better appreciate the significance of thorough research and analysis in their respective areas of interest.
Introduction to Research and Analysis
Research and analysis encompass a systematic approach to collecting and interpreting information. Research is defined as the methodical process of gathering data to answer a specific research question or solve a problem. This could involve various research methods, including surveys and qualitative content analysis. Analysis, on the other hand, refers to the breakdown of complex data into manageable parts, allowing researchers to analyze data effectively. Together, these processes form the backbone of effective decision-making and knowledge acquisition.
Definition of Research and Analysis
To truly understand research and analysis, one must recognize that research involves the systematic collection, analysis, and interpretation of information. This process begins with identifying a research question, followed by designing a study that incorporates appropriate data collection techniques. Once the data is gathered, researchers employ various analysis methods to assess the information. Analysis is used to attain deeper insights, transforming raw data into meaningful conclusions. By understanding these definitions, we can appreciate the nuances of data analytics in different contexts.
The Importance of Analysis in Research
Analysis plays a critical role in research as it provides the context needed for informed decision-making. Effective analysis transforms raw data into actionable insights, allowing decision-makers to assess various scenarios and their potential impact. Without sound analysis, choices may be made based on incomplete or misleading information, leading to poor outcomes. This emphasizes the necessity of using analytical techniques to quantify and evaluate data accurately. In essence, analysis in research not only clarifies findings but also opens doors to recognizing opportunities for improvement.
Roles of an Analyst in Research
An analyst’s role in research is pivotal, as they are responsible for interpreting data and providing insights that inform decision-making processes. Utilizing various analytical techniques, including statistical methods and data modeling, analysts uncover patterns within the data. Their expertise allows them to conduct research that bridges the gap between data collection and actionable insights. Critical thinking skills are essential for analysts, enabling them to evaluate data analytics effectively and draw meaningful conclusions that can significantly impact research outcomes. In today’s digital age, tools like VPN Unlimited can ensure secure and private access to data, which is crucial for maintaining the integrity of research processes.
Fundamentals of Data Analysis
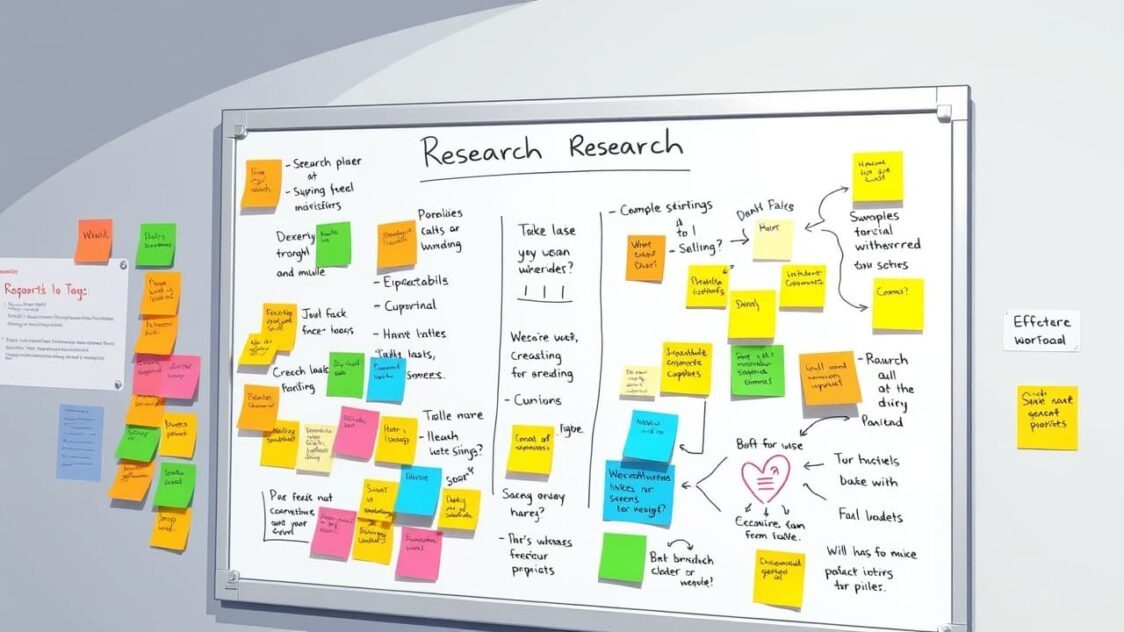
Types of Data Analysis
Data analysis can be categorized into several types, including descriptive, inferential, predictive, and prescriptive analysis. Descriptive analysis summarizes historical data to understand what has happened, allowing researchers to assess trends over time. Inferential analysis uses a random sample to make inferences about a population, providing insights that can extend beyond the immediate dataset. Predictive analysis forecasts future outcomes based on historical data, which is particularly useful in public health for anticipating disease outbreaks. Meanwhile, prescriptive analysis recommends actions based on data analysis findings, guiding decision-makers in selecting optimal strategies. Each type serves a distinct purpose, helping analysts conduct research that drives informed decisions based on the nature of the data being analyzed.
Tools and Techniques for Effective Data Analysis
Effective data analysis requires various tools and techniques, including statistical software, data visualization tools, and data mining techniques. Commonly used software such as R, Python, and SPSS empower analysts to automate processes involved in data manipulation and statistical analysis. Data visualization tools like Tableau and Power BI play a crucial role in presenting data in an easily digestible format, enabling stakeholders to grasp complex information quickly. Techniques such as regression analysis, clustering, and machine learning algorithms are employed to uncover patterns and relationships within the data. By combining these tools and techniques, analysts can enhance their data analytics capabilities, transforming data collection into actionable insights that inform research methodologies.
Common Challenges in Data Analysis
Data analysis can be fraught with challenges, including data quality issues, lack of standardization, and difficulties in interpreting results. Incomplete or inaccurate data can lead to misleading conclusions, making data cleaning and validation essential steps in the analysis process. Analysts often face the challenge of integrating data from multiple sources, which may have different formats and structures. This integration is vital to conduct research effectively, as it allows for a comprehensive view of the data landscape. Additionally, effectively communicating complex analytical results to non-technical stakeholders can be a significant hurdle. Analysts must present findings in a clear and concise manner, ensuring that all parties understand the implications of the analysis in research and how it impacts decision-making processes.
Conducting Effective Surveys as a Research Method
Designing Your Survey for Optimal Results
Designing an effective survey is a crucial step in conducting research and analysis, as it directly impacts the data collection process and the quality of the insights gathered. To achieve optimal results, researchers must carefully consider their research objectives and the target audience when formulating questions. Surveys should include a variety of question types—such as multiple-choice, Likert scale, and open-ended questions—to capture a broad spectrum of data. This mix allows analysts to explore both qualitative and quantitative aspects of respondents’ opinions. Moreover, pre-testing the survey with a small group can uncover potential issues, facilitating refinements that enhance clarity and engagement. Ensuring anonymity and confidentiality is equally important, as it encourages participants to provide honest and candid responses, ultimately leading to more accurate data analysis.
Analyzing Survey Data
Once the survey is administered, analyzing survey data becomes the next critical phase in the research methodology. This process involves several key steps, including data cleaning, coding responses, and applying various statistical analysis techniques. Data cleaning ensures that the collected dataset is free from errors and inconsistencies, which is paramount for reliable analysis. Coding, on the other hand, transforms qualitative responses into quantifiable data, enabling analysts to assess patterns and trends effectively. Utilizing descriptive statistics provides a summary of the data, while inferential statistics allows researchers to draw conclusions about the broader population based on the sample. Visualization techniques, such as charts and graphs, are essential tools that present findings in an accessible format, making it easier for stakeholders to understand and act upon insights derived from the data.
Case Studies of Successful Survey Analysis
Case studies of successful survey analysis illustrate the profound impact that well-designed surveys can have on decision-making processes. For example, a company may conduct a customer satisfaction survey to gather insights into areas needing improvement in their service delivery. By analyzing the survey results, analysts can identify trends that inform strategic decisions, such as enhancing customer support services or adjusting product offerings to better meet client needs. Another compelling example is a public health survey that uncovers community health requirements, leading to targeted interventions and optimized resource allocation. These case studies underscore the importance of surveys as a powerful research technique and highlight the value of effective data analysis in deriving actionable insights that can significantly influence outcomes in various fields, including public health and market research.
Advanced Techniques in Research and Analysis
Qualitative vs. Quantitative Analysis
Qualitative and quantitative analysis are two fundamental approaches in research, each serving distinct purposes. Qualitative analysis focuses on understanding the underlying reasons and motivations behind behaviors, often utilizing methods such as interviews, focus groups, and content analysis. It provides rich, contextual insights but may lack generalizability. In contrast, quantitative analysis involves the statistical examination of numerical data to identify patterns, relationships, and trends. This approach allows for broader generalizations but may overlook the depth of individual experiences. Combining both methods can provide a comprehensive understanding of research questions.
Using Statistical Methods to Enhance Analysis
Statistical methods play a crucial role in enhancing the analysis process, allowing researchers to draw reliable conclusions from data. Techniques such as regression analysis, hypothesis testing, and ANOVA help analysts understand relationships between variables and assess the significance of their findings. Utilizing statistical software can streamline the analysis process, enabling more complex calculations and visualizations. By applying these methods, analysts can provide evidence-based insights that support decision-making and validate research hypotheses, ultimately improving the quality of analysis in research and enhancing overall outcomes.
Integrating Data Analysis with Other Research Methods
Integrating data analysis with other research methods, such as case studies or ethnography, can enrich the overall research process. By combining quantitative data with qualitative insights, researchers can develop a more holistic understanding of the research topic. For example, a mixed-methods approach may involve conducting a survey to gather numerical data and then following up with interviews to explore the reasons behind the survey responses. This integration allows for triangulation of data, enhancing the credibility and depth of the findings while providing a comprehensive view of the research question.
Interpreting Results and Making Recommendations
Best Practices for Result Interpretation
Interpreting results requires careful consideration of the context and the implications of the findings. Analysts should avoid overgeneralizing results and consider the limitations of the data. Best practices include validating findings through triangulation, seeking peer feedback, and ensuring that interpretations align with the research objectives. It’s essential to communicate results clearly and transparently, highlighting both strengths and weaknesses of the analysis. Providing actionable insights based on the findings can help stakeholders make informed decisions and enhance the impact of the research conducted.
How to Present Findings Effectively
Effectively presenting findings is crucial for ensuring that insights are understood and acted upon. Analysts should use clear and concise language, avoiding jargon that may confuse stakeholders. Visual aids, such as charts, graphs, and infographics, can enhance comprehension and retention of information. Structuring presentations logically, starting with key findings followed by supporting data, can guide the audience through the analysis. Engaging the audience through interactive elements, such as Q&A sessions or discussions, can further enhance the presentation’s impact and facilitate a deeper understanding of the analysis in research.
Making Data-Driven Recommendations
Making data-driven recommendations involves synthesizing the analysis results into actionable steps for stakeholders. Analysts should ensure that recommendations are grounded in the data and aligned with the research objectives. It’s important to consider the feasibility and potential impact of each recommendation, providing a rationale for why specific actions should be taken. Presenting recommendations alongside potential risks and benefits can help decision-makers weigh their options effectively. By framing recommendations in the context of the broader research findings, analysts can enhance their persuasiveness and relevance, ultimately influencing effective decision-making.